2024 Achievements
Note: building OpenAI projects for classic Sega 8/16-bit video games integration is a big achievement! |
2025 Objectives
|
Artificial Intelligence
Artificial Intelligence refers to capability of machines to imitate human intelligence. AI empowers machines to acquire knowledge, adapt and independently make decisions like teaching a computer to act human like.
Machine Learning
AI involves a crucial element known as Machine Learning. ML is akin to training computers to improve tasks without providing detailed instructions. Machines utilize data to learn and enhance the performance without explicit programming and concentrates on creating algorithms for computers to learn from data to improve.
Deep Learning
Deep Learning involves artificial neural networks inspired by the human brain: mimicking how human brains work. DL excels at handling complex tasks and large datasets efficiently and achieves remarkable success in areas like natural language processing and computer vision despite complexity and interpretation challenges.
Generative AI
Generative AI is the latest innovation in the AI field. Instead of just identifying patterns GenAI goes one step further by actually attempting to produce new content that closely resembles what humans might create.
Outline
Artificial Intelligence | Artificial Intelligence is the "big brain" |
Machine Learning | Machine Learning is its learning process |
Deep Learning | Deep Learning is its intricate wiring |
Generative AI | Generative AI is the creative spark |
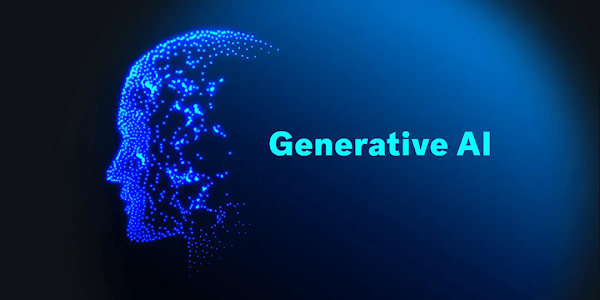
Gen AI and LLMs are revolutionizing our personal and professional lives From supercharged digital assistants to seemingly omniscient chatbots these technologies are driving a new era of convenience, productivity, and connectivity.
Traditional AI uses predictive models to classify data, recognize patterns, + predict outcomes within specific context whereas Gen AI models generate entirely new outputs rather than simply making predictions based on prior experience.
This shift from prediction to creation opens up new realms of innovation: in healthcare traditional predictive model can spot suspicious lesion in lung tissue MRI whereas GenAI could also determine the likelihood that patient will develop pneumonia or other lung diseases and offer treatment recommendations based on best practices gleaned from thousands of similar cases.
Example
GenAI powered healthcare chatbots can assist patients and healthcare providers and medical administrators:
01. Symptom Checker | 07. Mental Health Support |
02. Appointment Scheduling | 08. Insurance and Billing Assistance |
03. Medication Reminders | 09. Virtual Consultations and Telemedicine |
04. Health Tips and Preventive Care | 10. Health Records Access |
05. Lab Results Interpretation | 11. Emergency Triage |
06. Chronic Disease Management |
By leveraging conversational AI healthcare chatbot can improve patient engagement and provide real-time support and optimize the workflow for healthcare providers. Finally, Reinforcement Learning From Human Feedback RLHF can be integrated to further improve model performance over original pre-trained version!
Future
Artificial Intelligence is changing industries across the globe from healthcare and finance to marketing and logistics. As we enter 2025, the demand for skilled AI professionals continues to soar. Start out by building strong foundations in Python and understand key concepts such as machine learning and neural networks.
Therefore, whether an AI beginner or seasoned tech professional, here are the top 10 AI skills for success:
No. | AI Skill | Key Tools |
01 | Machine Learning (ML) | Scikit-learn, TensorFlow, PyTorch |
02 | Deep Learning | Keras, PyTorch, Google Colab |
03 | Natural Language Processing (NLP) | NLTK, SpaCy, GPT-based models (e.g., GPT-4) |
04 | Data Science and Analytics | NumPy, Pandas, Jupyter Notebooks |
05 | Computer Vision | OpenCV, YOLO (You Only Look Once), TensorFlow |
06 | AI Ethics and Bias Mitigation | AI Ethics Courses, Fairness Indicators (Google) |
07 | AI Infrastructure and Cloud Computing | Amazon Web Services, Microsoft Azure, Google Cloud AI |
08 | Reinforcement Learning | OpenAI Gym, TensorFlow Agents, Stable Baselines3 |
09 | AI Operations (MLOps) | Docker, Kubernetes, Kubeflow, MLflow |
10 | Generative AI | Generative Adversarial Networks, DALL-E, GPT models |
Finally, the GenAI market is poised to explode, growing to $1.3 trillion over the next 10 years from market size of just $40 billion in 2022. Therefore, it would be extraordinary to integrate GenAI to build content for OpenAI-based retro video games only to be trained by Reinforcement Learning algorithms to beat them J